Table of Contents
The binomial distribution is a discrete probability distribution commonly used in statistics. It represents the probability of achieving exactly ‘x’ successes in ‘n’ independent trials where each trial has a fixed probability of success ‘p’. In contrast, the normal distribution is continuous. This article discusses the Binomial Distribution in detail.
What is Binomial Distribution?
The binomial distribution is a statistical distribution that describes the probability of obtaining a specific number of successes in a fixed number of independent trials each with the same probability of success. It provides insight into how likely a particular outcome is when each trial results in one of two possible outcomes (success or failure).
Features of Binomial Distribution
- In binomial distribution, each trial results in one of two possible outcomes – success or failure.
- In reference to the concept of binomial distribution, the probability of success remains the same across all trials. If the probability of success is p, the probability of failure is q = 1 − p.
- Each trial in binomial distribution is independent. It means that the outcome of one trial does not influence the outcome of another.
- The binomial distribution is discrete, meaning it deals with countable outcomes, unlike the continuous normal distribution which deals with a range of values.
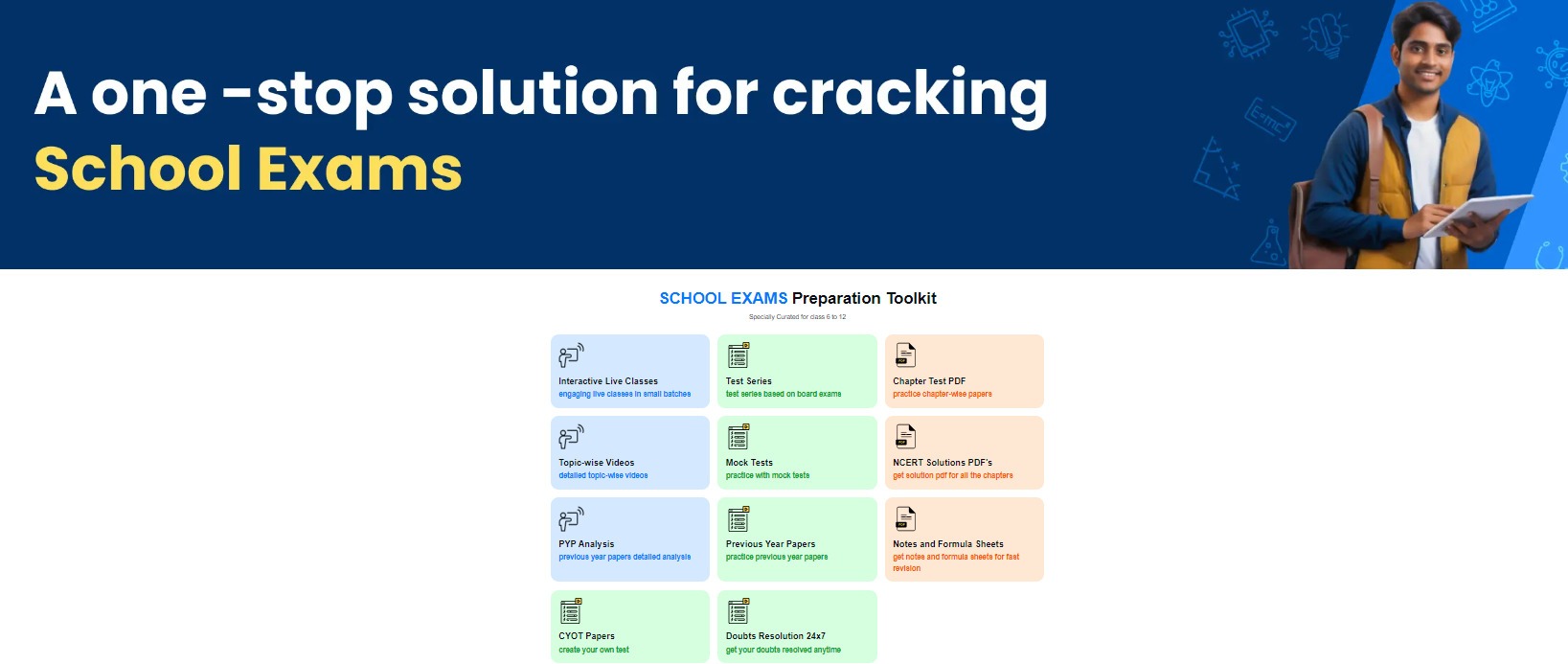
Binomial Distribution Formula
The binomial distribution provides the probability of achieving exactly x successes in n independent trials, each with a probability of success p. The formula for the binomial distribution is:
P(x: n, p) = nCx px(1 – p)n – x
Or
P(x: n, p) = nCx px(q)n – x
Where:
- The number of Trials (n) represents the total number of experiments or trials.
- The number of Successes (x) represents the number of successful outcomes desired.
- Probability of Success (p) represents the probability of success on a single trial.
- Combination (nCx) represents the number of ways to choose x successes from n trials without regard to order. Mathematically, it is given by: nCx = n! / (x! (n – x)!)
- where n! denotes the factorial of n.
Why Binomial Distribution is Important?
The binomial distribution is a discrete probability distribution that summarises the likelihood of a particular number of successes in a fixed number of independent trials, each with the same probability of success. It is widely used in statistics to model binary scenarios where there are only two possible outcomes (success or failure). It is used in experiments where outcomes are mutually exclusive, such as flipping a coin, quality control testing, or predicting the success rate of a new drug. Summarising the chances of different success counts in limited trials provides valuable insights into decision-making processes that involve risk and probability.
Fun Facts About Binomial Distribution
- The binomial distribution is widely used in social sciences, particularly in modelling dichotomous outcomes such as predicting election results and estimating probabilities like whether an individual will survive a certain period.
- Beyond social sciences, it also finds applications in finance, banking, and insurance, where binary outcomes like defaults, claims, or market movements are analysed using binomial probability.
- The expected value or mean of a binomial distribution can be calculated using the formula μ = n × p, where n is the number of trials and p is the probability of success. This represents the average number of successes expected in the trials, making it a critical parameter for statistical analysis and decision-making.
Binomial Distribution – Case Studies
Coin Tossing Example: If a fair coin is tossed 100 times, the probability of getting heads in each toss is ½ or 0.5. Using the binomial distribution, the expected number of heads can be calculated as:
Expected Value = n × p = 100 × 0.5 = 50
This means that on average, you would expect to get 50 heads out of 100 tosses.
Basketball Free Throws Example: Consider a basketball player making free throws, where each successful shot is represented by 1 (success) and each miss by 0 (failure).
If the probability of making a free throw is p, the binomial distribution can be used to calculate the probability of a certain number of successful free throws out of n attempts.
Therefore, we can formulate a binomial distribution function as follows:
P(x: n, p) = nCx px(1 − p)n − x = nCx px(q)n − x
Where:
- The number of Trials (n) represents the total number of experiments or trials.
- The number of Successes (x) represents the number of successful outcomes desired.
- Probability of Success (p) represents the probability of success on a single trial.
- Combination (nCx) represents the number of ways to choose x successes from n trials without regard to order. Mathematically, it is given by: nCx = n! / (x!(n − x)!), where n! denotes the factorial of n.
Statistical Properties of Binomial Distribution
Average (Mean): The average or mean of a binomial distribution is calculated as:
Mean = μ = n × p
where n is the number of trials and p is the probability of success in each trial.
Variance: The variance of a binomial distribution is given by:
Variance = σ2 = n × p × (1−p)
This measures the spread or dispersion of the distribution.
Symmetry and Skewness:
- Symmetric Distribution: When the probability of success is p = 0.5, the binomial distribution is symmetric around its mean. This is similar to flipping a fair coin where the chances of heads or tails are equal (50% each).
- Left Skewed: When p > 0.5, the distribution becomes left-skewed, meaning it has a longer tail on the left side of the mean.
- Right Skewed: When p < 0.5, the distribution becomes right-skewed, with a longer tail on the right side of the mean.
Applications of Binomial Distribution
- Finance:
- Borrower Default Risk: Banks use binomial distribution to estimate the probability of a borrower defaulting on a loan. This helps in determining lending policies and managing risk.
- Loan Amounts and Reserves: It aids in deciding how much money to lend and the amount to keep in reserve to cover potential defaults.
- Insurance:
- Premium Estimation: Insurance companies apply the binomial distribution to estimate the probability of claims and set insurance premiums accordingly.
- Risk Assessment: It helps in evaluating the likelihood of various types of insurance claims and managing overall risk.
- Other Fields:
- Medical Research: Used to determine the effectiveness of treatments by analysing success rates in clinical trials.
- Quality Control: Applied in manufacturing to assess the probability of defects in a batch of products.
Binomial Distribution FAQs
What is a binomial distribution?
The binomial distribution is a statistical model that shows the probability of getting a certain number of successes in a fixed number of trials with the same probability of success.
How do you calculate binomial distribution?
To calculate the binomial distribution we use the formula: P(x: n, p) = nCx px(1 - p)n - x = nCx px(q)n - x where n is the number of trials, x is the number of successes, and p is the probability of success.
Where is the binomial distribution used?
The binomial distribution is used in finance, insurance, and research to model scenarios with two possible outcomes like predicting loan defaults or evaluating treatment success.